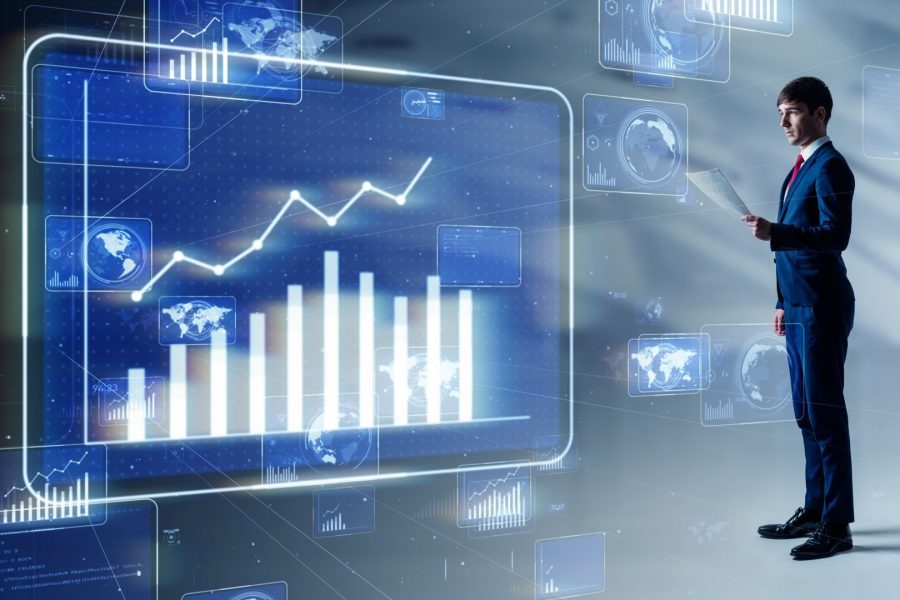
How artificial intelligence can facilitate processes for companies and their customers is the subject of a collaboration between the University of Salzburg (Machine Learning/Department of Computer Science and Statistics/Department of Mathematics) and Porsche Holding Salzburg. We provide data that students then embed into prediction, optimisation or pattern recognition issues. One of these is Sebastian Zeng, who presents his research project to us in the following interview.
Sebastian, your project involves time series analysis. What exactly did you investigate?
In science and industry, decisions constantly have to be made in terms of selecting suitable methods for process optimisation. This is also the case at Porsche Holding: here, the question arises, for example, as to the right tools for predicting the demand for spare parts, for forecasting the number of visitors in a dealership, or for measuring the influence of historical events on liquidity planning.
I investigated methods that can derive suitable probability forecasts for this on the basis of historical data. Time series analysis is a relatively well-researched field of science, and numerous traditional approaches exist that are based on purely statistical models. For some time, however, machine learning methods have achieved remarkable progress. Nevertheless, developing an interpretable model for generating accurate probability forecasts remains a challenge – this is the task I set myself.
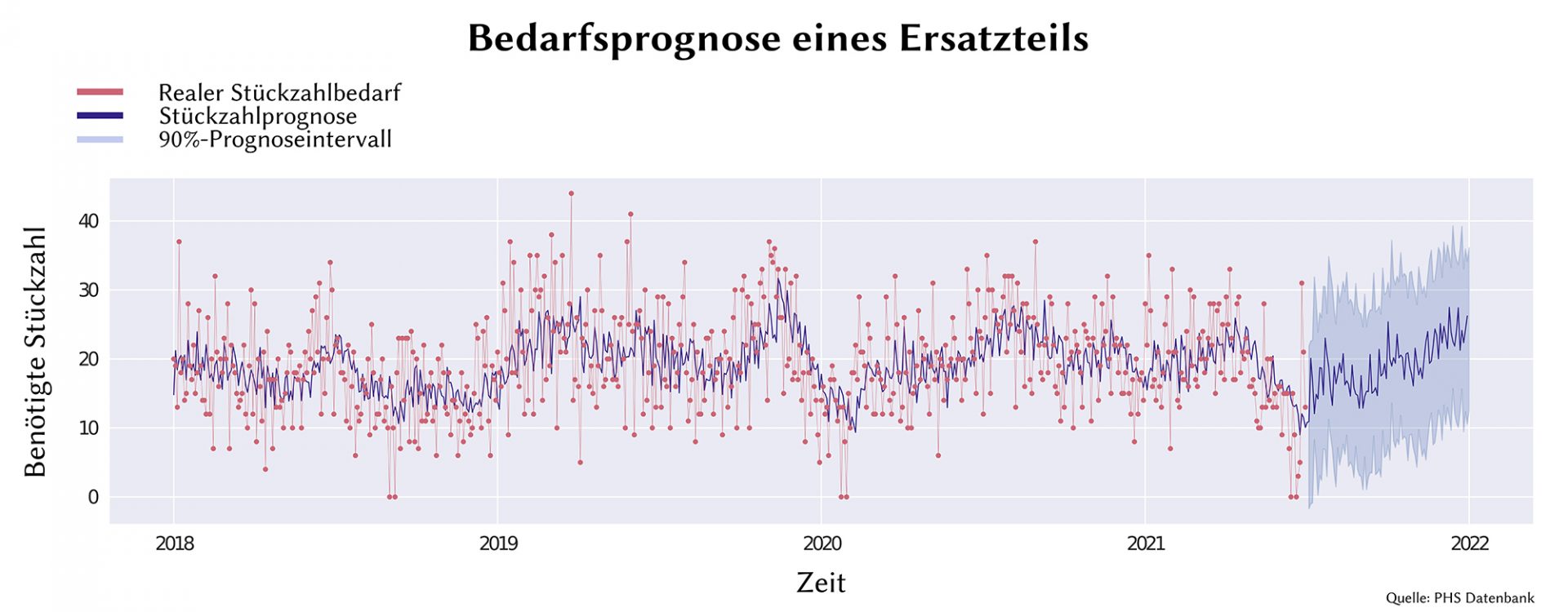
A classic use case for time series analysis at Porsche Holding: the predicted demand for spare parts
How did Porsche Holding support you in your study?
With the synergy of economic and scientific interests, the collaboration between the Department of Computer Science at the University of Salzburg and Porsche Informatik puts the focus on a number of interesting topics. With the support of the Data-Driven Business Solutions team at Porsche Informatik, I decided to study time series analysis for my doctorate, which is both an exciting research topic and very useful in terms of its application.
Modern solutions for the development of time series analysis models need to be flexible with respect to the data source. This means that the analysis model should calculate universally reliable forecasts, regardless of the data set and the application area. It has to be assumed that the volume of data processing will continue to increase in the future. It therefore needs to be possible to process a large number of time series simultaneously.
Porsche Informatik provided me with comprehensive data from various fields of application within Porsche Holding Salzburg. We discussed these in detail at regular meetings and discussed questions concerning content and processing. The support of the team with regard to data truncation and in developing manageable sub-targets for achieving a reliable database was particularly helpful, while the DBS team also supported me with its know-how concerning the automotive industry.
What is the result of your work?
The aim of my research was to develop a new model for time series analysis that is based on modern approaches from the fields of deep neural networks, machine learning and topological data analysis. As I already mentioned before, models based on these methods provide better results than purely statistical ones. Many of the solutions can be executed by users without any expertise in time series analysis and show advantages in terms of the processing of large databases.
I also integrated mathematical theories from topological data analysis, a field of research that deals with the structural analysis of geometric objects and quantifies this information. The results obtained from this are incorporated into my forecast model in addition to the raw data and thereby improve the forecast.
As a result, I have developed a model that integrates these ideas, which was previously unpublished in this form and provides excellent state-of-the-art prediction results. Following extensive empirical comparisons, I submitted my work for scientific publication in May 2021.
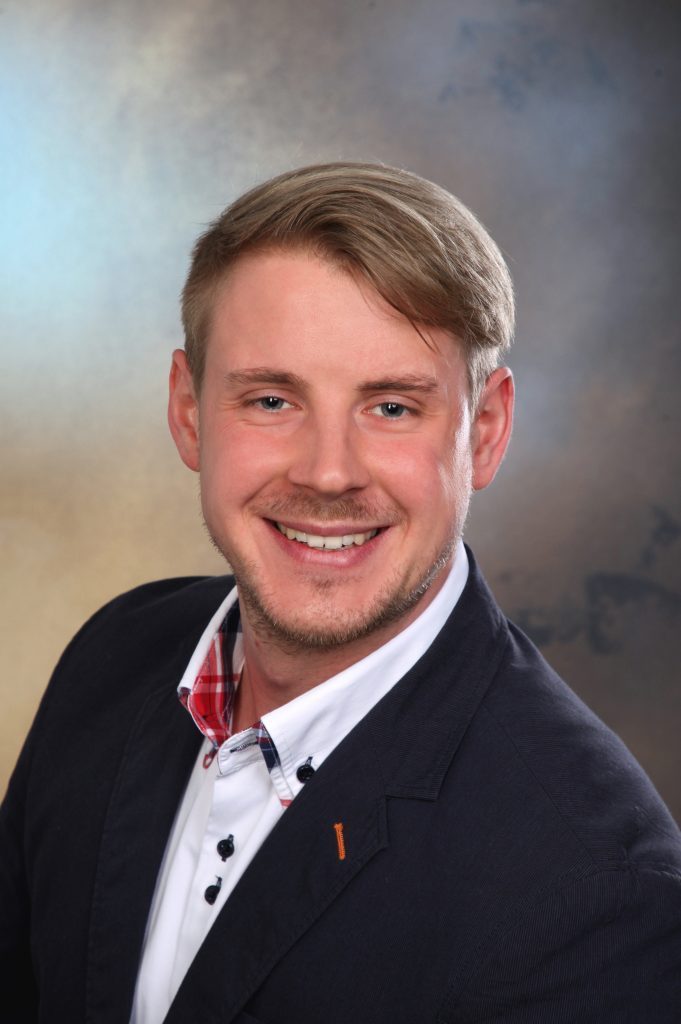
Sebastian Zeng, time series researcher
What are the specific benefits of the results of your work? How can they support Porsche Holding in the automotive industry, for example?
Following this successful research into new methods, I now want to evaluate the approaches and model variants that Porsche Holding and Porsche Informatik are currently using in collaboration with the DBS team. We will then compare these results with those based on my developments. If the quality of the forecasts improves – which is what I expect – these algorithms will be integrated into the Porsche Holding data lake and be used in Porsche Holding’s products. The university will additionally support this by providing programme code libraries and assisting with questions on functionality.